Building RAG in Ruby, using BAML, with streaming!
How to do RAG with Ruby streaming AI APIs
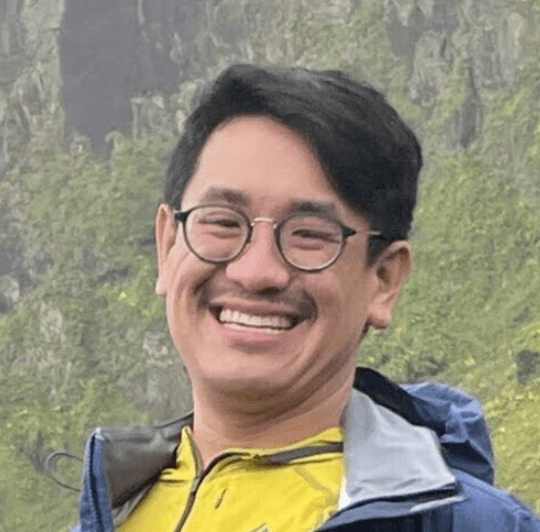
Sam Lijin
LinkedInThis article will walk you through implementing RAG with citations in Ruby using BAML to:
- get structured outputs from LLMs
- stream partially structured output back, as it's being generated
The final example looks like this:
The source code is available on Github
This project uses BAML, our prompt configuration language that extends Jinja to help you get structured outputs from LLMs. BAML can autogenerate a Ruby client that can call our prompt templates, handle all deserialization, and stream the results. More on this below!
1. Initialize BAML
We will use the BAML prompt templating configuration to build our RAG prompt.
bundle init
bundle add baml
bundle exec -- baml-cli init
- this will create a directory to place .baml files in.- Get the VSCode extension for BAML syntax highlighting and playground capabilities.
2. Build a prompt
In BAML you build prompts using a schema-first approach. What's this mean? Well, prompt templates are basically functions that take in input variables and return structured outputs. In BAML you literally define your function signatures and then write the prompt using that signature, which helps reduce prompt engineering.
In this RAG example need a function that takes in a question and a list of documents, and outputs an Answer
object, with citations, so this is the function we will build:
function AnswerQuestion(question: string, context: Context) -> Answer
Define your input variables
Lets declare the Context
input type in a .baml file in the project, which we'll use to pass our documents to the LLM:
class Context {
documents Document[]
}
class Document {
title string
text string
link string
}
Define the Answer schema
The output schema is an Answer
object that contains a list of citatons.
When humans write a paper, they usually cite other papers and then write out their answer using those citations. We will make the LLM do the same:
- First give us the citations from the documents that answer the question
- Then give us the answer
This will help reduce hallucinations since the LLM will be more likely to use the generated citations in the answer. You can change this order if you want to experiment with different approaches.
Here's the answer schema, which you can add to any .baml file in your project:
class Answer {
// note this is first in the answer schema -- the LLM will need to gather citations first!
answersInText Citation[]
answer string @description(#"
When you answer, ensure to add citations from the documents in the CONTEXT with a number that corresponds to the answersInText array.
"#)
}
class Citation {
documentTitle string
sourceLink string
relevantTextFromDocument string
number int @description(#"
the index in this array
"#)
}
Now we have the full function signature types! Let's build the prompt.
Build the LLM function
Here's the full function syntax in BAML -- which will get translated into a python or typescript function.
BAML prompts use the Jinja templating language to help you write structured prompts. You can use Jinja to loop over lists, conditionally render parts of the prompt, and more. We added a couple of helper functions to Jinja, explained below.
function AnswerQuestion(question: string, context: Context) -> Answer {
client GPT4 // you can use anthropic, openai, ollama, and others. See the BAML documentation for more.
prompt #"
Answer the following question using the given context below.
CONTEXT:
{% for document in context.documents %}
----
DOCUMENT TITLE: {{ document.title }}
{{ document.text }}
DOCUMENT LINK: {{ document.link }}
----
{% endfor %}
{{ ctx.output_format }}
{{ _.role("user") }}
QUESTION: {{ question }}
ANSWER:
"#
}
There are 2 predefined BAML macros -- ctx.output_format
and _.role("user")
-- to help us write the output schema instructions into the prompt, and mark a specific part of the prompt as a "user" message.
The {{ ctx.output_format }}
line will be replaced with the output structure instructions at runtime, which uses your @description
annotations to make things clearer to the LLM.
Answer using this JSON schema format:
{
answersInText: [
{
documentTitle: string,
sourceLink: string,
relevantTextFromDocument: string,
// the index in this array
number: int,
}
],
// When you answer, ensure to add citations from the documents in the CONTEXT with a number that corresponds to the answersInText array.
answer: string,
}
BAML uses type definitions in prompts, which are 4x less costly than JSON Schema.
If you use the BAML Playground in VSCode you can see it rendered in real-time, so you don't have to guess what string will be sent to the LLM client at runtime:
Stream your BAML-defined function in Ruby
When you save a .baml file, the BAML VSCode extension generates a Ruby client to call the function. Everything runs in your own machine.
Using this generated client, we can write our own code to handle this:
require_relative "baml_client/client"
Baml.Client.stream.AnswerQuestion(question: input, context: Baml::Types::Context.new(documents: DOCUMENTS)).each_with_index do |partial_answer|
puts partial_answer.inspect
end
Note that for the purpose of this tutorial we are not addressing how to do document chunking, or doing similarity search. We are just using a hardcoded list of documents.
Render the answer and citations
Now that we have our streaming, we can use the fact that BAML is parsing partial_answer
into an instance of Baml::PartialTypes::Answer
for us:
require_relative "baml_client/client"
Baml.Client.stream.AnswerQuestion(question: input, context: Baml::Types::Context.new(documents: DOCUMENTS)).each_with_index do |partial_answer|
answer = <<~ANSWER
Answer:
#{partial_answer.answer}
Citations:
#{citations}
(meta: #{i + 1} stream events received)
ANSWER
puts answer
end
And you're done!
You should now be able to stream responses. Feel free to change the prompt according to your needs. The VSCode BAML playground makes it very easy to test your prompt on various test cases without having to run the whole program.
With BAML we were able to just call a function without worrying about
- Retries, redundancy
- Deserialization (BAML fixes common issues with JSON output from LLMs as well, such as unescaped quotes or missing brackets)
- Partially parsing a stream of responses
and as we were prompt engineering using our types, we always got to see the full prompt.
The full source code is available on Github, along with some more examples.
You can check out the BAML Documentation for more examples, and join our Discord if you have any questions or need help!